How can we understand the demographics of riders across our system, to make equitable transit decisions?
Depending on resources available, there are multiple methods transit agencies can use to try to estimate their ridership demographics, both to comply with Title VI and supporting policies and to make better decisions about their system regarding equity. A previous post, Does U.S. Census Data Predict MBTA Bus Ridership?, summarizes a project which compared the two most common ways transit agencies can estimate ridership for minority and low-income riders: either by conducting a passenger survey to collect demographic information from a sample of riders or using U.S. census data to estimate ridership based on the residential demographics of surrounding stops and routes. Conducting a passenger survey has been – and as confirmed by this blog post is still – the preferred method to understand rider demographics. However, the MBTA continues to use census data as an estimate for certain applications, as it is more easily accessible and less resource-intensive compared to the rider census survey.
A new method to address this issue might be found in StreetLight Data, a “big data” platform that offers location-based services (LBS) data through anonymized location records from smartphones and navigation devices. Using StreetLight’s traveler demographics, which are sourced from census data, this new tool could change the way the MBTA factors rider demographics into its decisions. In the past, when using census data, ridership demographics have been estimated in terms of residential areas around service stops and routes. However, with access to new data and tools like StreetLight, we can improve upon this rudimentary use of census data to understand the demographics of riders using our service based on actual travel patterns. This raises the ultimate question we are exploring:
Is there a difference between the demographic composition of certain zones in our service area vs. the demographic makeup of the travelers traveling to, from, or through those zones?
StreetLight Analyses
Using the StreetLight platform, one can perform Zone Activity analyses to get the volume of trips that start in, end in, or pass through specified zones of interest. With the Home Work Location Add-On, StreetLight also offers the home location of the people making these trips. With this additional data, we can abstract the actual block groups travelers live in which give us more accurate estimates of their demographics. To evaluate our research question, we set up analyses to investigate the ‘All Vehicle’ trips from 2021 made to, from, and through block groups in the MBTA service area. Looking at all vehicle trips allowed us to capture riders’ actual travel patterns, not just how they can travel on our system.
Resident-made Trips
Conducting our analyses gave us a table with the volume of trips made to, from, and through each block group for three categories of travelers: Residents, Workers, and Visitors. This data allowed us to first investigate what percentage of trips in each block group were made by residents. Doing this first was important because if block groups had high resident-made trips, there would be little demographic difference between riders and residents to examine and the aforementioned use of census data would be unproblematic.

The graphic to the left shows the distributions of the resident-made trip percentages for trips starting, ending, or passing through each service area block group.
For trips starting and ending in each zone (shown in the top two graphs), the block groups had an average of 35% resident-made trips. However, if you look at the third graph, which captures the trips that pass through each zone, the block groups had a much lower resident-made trip average of 5%. This explains why if you look at the bottom-most graph, which shows the average among all trip types you can see the distribution skews lower, averaging closer to 24% resident-made trips for each block group.
These results make sense, as we would expect a higher proportion of residents to be starting and ending their trips in their home block groups – this is what our typical use of census data assumes. Yet, the results give us insight into the groups of travelers we are missing when we make this assumption. The first group is those ~65% of people who are neither starting nor ending their trips in their home block groups. The second group of people is those travelers passing through block groups as a part of their larger journey. Without considering these trips, we could be discounting areas most utilized by travelers simply because they are not popular origins/destinations.
Zone vs. Traveler Demographics
With all the data received from StreetLight we hoped to build a table which could be used to compare the demographic composition of block groups, as well as the average demographics of people making trips within these block groups. The analyses results provided a table summarizing the home block group locations of travelers making trips made to, from, and through each zone for two categories of travelers: workers and visitors.
The below table is a sample of this.
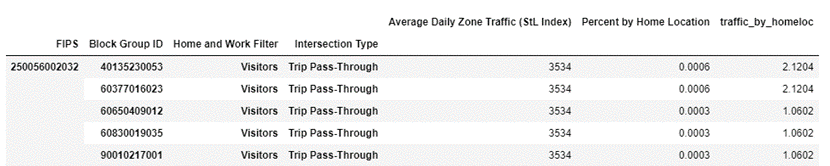
We were able to join census data from the American Community Survey 5-Year Data (2014-19) to this table to get a summary table that describes the demographics of the zones as well as travelers’ home block groups. From this, we estimated the volumes of low-income and minority travelers from each home block group (by traveler and intersection type). We then summed the traveler volumes by block groups to get a table with a row for each service area block group with the percentage of low-income and minority population living within that block group, as well as the percentage of these same populations traveling within these block groups.
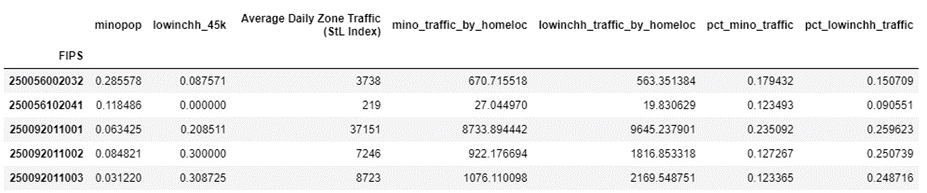
As an initial way to visualize the demographic difference between block group residents and travelers, we plotted these two variables for both the minority (left) and low-income (right) populations.

For our exploration, the most significant areas to note on these maps are the light blue block groups, as these are the block groups with low minority/low-income populations, but high traffic from these two populations.
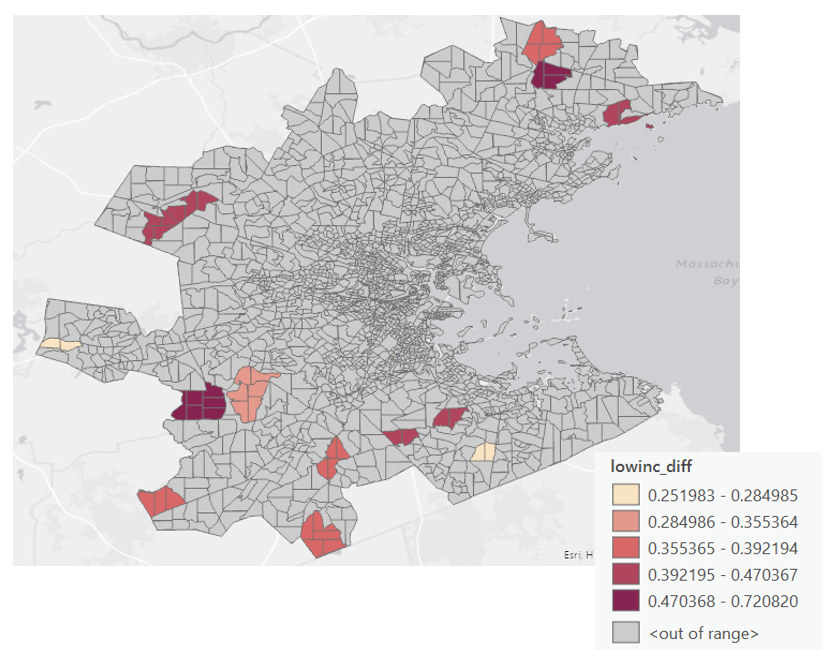
Taking a closer look at the data we were able to pinpoint block groups with low minority/low-income populations (less than 15%) but high traffic (more than 40%) from these two groups. These are the block groups that would be missed when using resident-based census data as a way to promote equitable transit. Notice the way the block groups are colored by the difference between these percentages, showing how residential-based census data could be underestimating minority and low-income travelers by 25% and up to 80%.

Conclusion
This blog post was motivated by the overarching question of how the MBTA can best utilize its resources to make decisions that promote fair and equitable transit access. This raises the question of how we can best estimate the demographics of riders across our services. One method, the rider census survey, gives an accurate estimate but is resource-intensive and hard to keep up to date with the volatile ridership trends. Another method, which uses residential-based demographics captured by census data to estimate ridership, is more easily accessible but makes the false assumption that travel on our system begins and ends at home for most riders. If we are to use census data as such, we must understand what this assumption does not capture: the difference between the demographic composition of the areas which our service runs through, and the actual demographics of the riders who utilize that service.
Based on this exploratory analysis, a difference appears to exist. With residential demographics underestimating traveler demographics by about 20%, using census data, however convenient, misses some populations of minority and low-income travelers. However, as demonstrated in the analyses performed to answer this research question, using new tools like StreetLight can allow us to consider this difference in the way we estimate riders’ demographics, giving us access to demographic data that is both easily accessible and accurate, empowering more equitable transit decisions. These data can also have interesting applications for municipalities and other entities that manage traffic by helping them determine and visualize how many vehicles are originating in their cities/neighborhoods, going to destinations in those places or simply passing through.
This data from Streetlight can be used anywhere we are currently using census data to predict the demographics of our ridership, allowing us to get rid of assumptions we previously have been forced to make. Ensuring that we provide fair service to groups that rely on our services the most can only be done if we increase or improve services that they use in their daily travel, not simply those that are closest to them.