Charts portray data in a visually appealing, easy to read format. This tends to hide the sometimes complex story behind their creation, particularly when using qualitative data. In this post, we will talk about qualitative data, and, using the sentiment by category chart created for the Fare Proposal Summary (PDF available at this link), we'll explore the journey of turning raw data to a finished form.
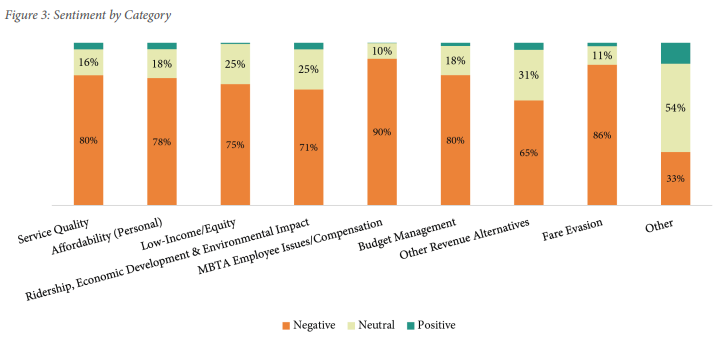
Qualitative Data
The MBTA collects and receives lots of data: the locations of our vehicles in time and space, every tap of a CharlieCard, results from passenger surveys. Some of the data is quantitative and (relatively) easily comparable. However, we also receive a lot of public feedback through twitter, emails, letters, phone calls, in the comment sections of surveys, and at public meetings.Making sense of all the feedback is more subjective than analyzing quantitative data. Addressing this subjectivity in methodical and structured ways allows us to find patterns and themes in the feedback and allows us to create a quantitative data set out of the qualitative feedback we receive. In order to explain our procedure this post will walk you through the creation of a single chart from the summary of public comment on the fare increase.
Thematic Analysis: Categorizing to Find Meaning
The journey of our chart began in January of 2016. From January 8th through February 12th, the MBTA solicited feedback regarding an upcoming fare increase. The MBTA collected more than 2,500 comments from an online comment tool, 11 public meetings, e-mail, phone and mail. Most of the comments came from the online comment tool, which gave passengers the ability to look up proposed fare and pass prices, answer a few questions, and leave a comment. E-mails were the second-largest category, followed by public meeting comments, phone calls, and letters.In order to properly analyze this data and to count each comment, we created representative categories of respondents' concerns. This is a key challenge when analyzing qualitative data. While categorizing numerical values is usually a straightforward process, this is not the case for qualitative information. In this case, more than 2,500 individuals gave their input, expressing a multitude of concerns, emotions, and ideas about the fare increase proposal. We decided to categorize the comments in two dimensions: the subject of the comment and the sentiment behind it.To refine our categories, several staff members independently read through a portion of the early feedback (approximately 500 comments) in order to find themes and patterns. The readers agreed that several topics appeared to matter greatly to our passengers. We used these topics to categorize the rest of the comments. These were:
- Service quality
- MBTA employee compensation and other concerns
- Budget management
- Other revenue alternatives
- Personal affordability
- Low-income/equity concerns
- Fare evasion
- Ridership, economic development and environmental impact
Not all comments were limited to one category (many people expressed multiple concerns), and not all comments submitted fit into these categories. There were many other categories that could have been used to organize the data. We believed that these categories encompassed the majority of the public's sentiment and fit the purpose of the report. As the analysis later showed, 155, or just 6% of the comments, did not fit any of the categories.
Categorizing by Sentiment
These categories, while helpful, did not fully express the information passengers wanted to convey, and their intent in leaving a comment. The additional dimension of “sentiment” allowed us to analyze both issues our passengers wanted the MBTA to target and their feelings towards the fare increase proposal. The sentiment dimension helps us differentiate comments that focus on the same subject (e.g. personal affordability), but provide opposite feedback (e.g. “I can't afford the fare!” versus “A ten-cent increase is not a problem”).We knew that this aspect of the analysis would be challenging. While it was easy to note a speaker's general sentiment during a public meeting, that was not the case for text comments. Deducing emotions from text is not always an accurate science, particularly when that text may contain an array of different emotions. With this in mind, we established rating criteria to make sure that sentiments were identified as consistently as possible. We also “spot-checked” a subset of comments for agreement by different readers. These checks showed that our ratings were acceptably consistent.
The Work Begins
Once the decisions to categorize by subject and sentiment were made, the truly laborious part of this process began. First, the raw data from different sources was cleaned and formatted in order to be analyzed. Then, the comments were divided into groups and given to a team of employees. They read every comment submitted to the MBTA, short or long, and categorized it. The depth and detail of information are the biggest benefits of qualitative data, but are also what makes it so time-consuming to analyze; a single equation cannot capture all the information being given.
Visualizing Results
We began to analyze the data as soon as all comments were categorized. We broke down the data in a variety of ways to identify the relationships between the categories and sentiments. We found that respondents' sentiment varied depending on the categories they mentioned. So, for our sentiment by category chart, we created a table detailing the total number of positive, neutral, and negative comments in each category. Sentiment seemed to vary between categories (although most categories were mostly negative).Since categories had different numbers of comments, the best way to present our topic of interest was to show the sentiment breakdown as a percentage of each category. This is best visualized as a percent stacked column chart. The chart allowed us to see passenger feelings towards each of the issues being brought up during the comment period, and how sentiment varied between categories.This chart, along with the other charts found in the Fare Proposal Summary Report, gives the MBTA a perspective on the issues that matter to the participants in the public comment process. The charts also give the public the opportunity to learn about what others are thinking. Qualitative data gives MBTA passengers a voice that can be measured and used by staff to make better-informed decisions.