Summary
As the COVID-19 pandemic emerged from the acute phase and vaccines became widely available in 2021, transit agencies like the Massachusetts Bay Transportation Authority (MBTA) faced a daunting challenge of drawing riders back into their systems. Despite system-wide staffing shortages, service disruptions, and ridership patterns that have yet to return to 2019 levels, ridership on the Commuter Rail has consistently outperformed the MBTA’s other services in the current COVID recovery era. In April 2021, as part of a multi-year vision to overhaul the system, the MBTA switched from a schedule focused on serving traditional peak-period (7 – 9 AM and 4:30 – 6:30 PM) commuters to providing steady, all-day service modeled on more legible “clockface” departures that happen at regular intervals throughout the day.
The MBTA’s effort to improve Commuter Rail service stems in part from a goal of supporting continued regional economic and population growth despite worsening vehicular congestion.1This change from service that prioritized work trips to more reliable hourly service was one step in a multi-year planning process known as MBTA Rail Vision 2020.1 In partnership with an independent advisory committee, MBTA leadership seeks to modernize the Commuter Rail system, prioritize mobility across the region, and improve service suitability for a wider array of travel patterns. As part of the efforts to reach these goals, Commuter Rail schedules were adjusted to be clockface departures, meaning that trains depart at regular, predictable intervals throughout the day. For example, inbound trains on the Needham Line leave Needham Heights station hourly, five minutes after the top of the hour. The clockface schedule changes on the Framingham/Worcester Line are detailed in Figures 1 – 2. The weekend schedule for this line was modified to align with consistent clockface departures, and added an additional train to the schedule. This scheduling change was a further step toward the goals for adapting the Commuter Rail service, after the launch of a $10, unlimited ride weekend pass in January 2019 by the T. In effect, the MBTA is shifting the Commuter Rail system toward a regional rail network, an approach which planners, activists, and policymakers have argued is essential to increasing ridership, balancing mode share, sustainably growing the regional economy, and providing more equitable access to the region.2,3
This post summarizes a forthcoming paper in Transportation Research Record that aims to determine the impacts on riders overall, and which subsets of riders were most affected in particular, more than two years after the implementation of clockface schedules. We used a regression analysis to see if improved clockface service impacted ridership recovery. We found that Commuter Rail outperformed other modes in ridership recovery, generating over 7,000 average daily weekday boardings and over 9,000 average weekend boardings. Further analysis demonstrated this schedule shift primarily benefited riders traveling for a variety of purposes, rather than just traditional office work. People in this group, to whom we refer to as general riders, are more likely to be low-income (household income below $75,000),young (25 or younger), and Hispanic. This research demonstrates the ability of all-day service on Commuter Rail to serve the MBTA’s key aims of increasing ridership and providing more equitable, accessible service.

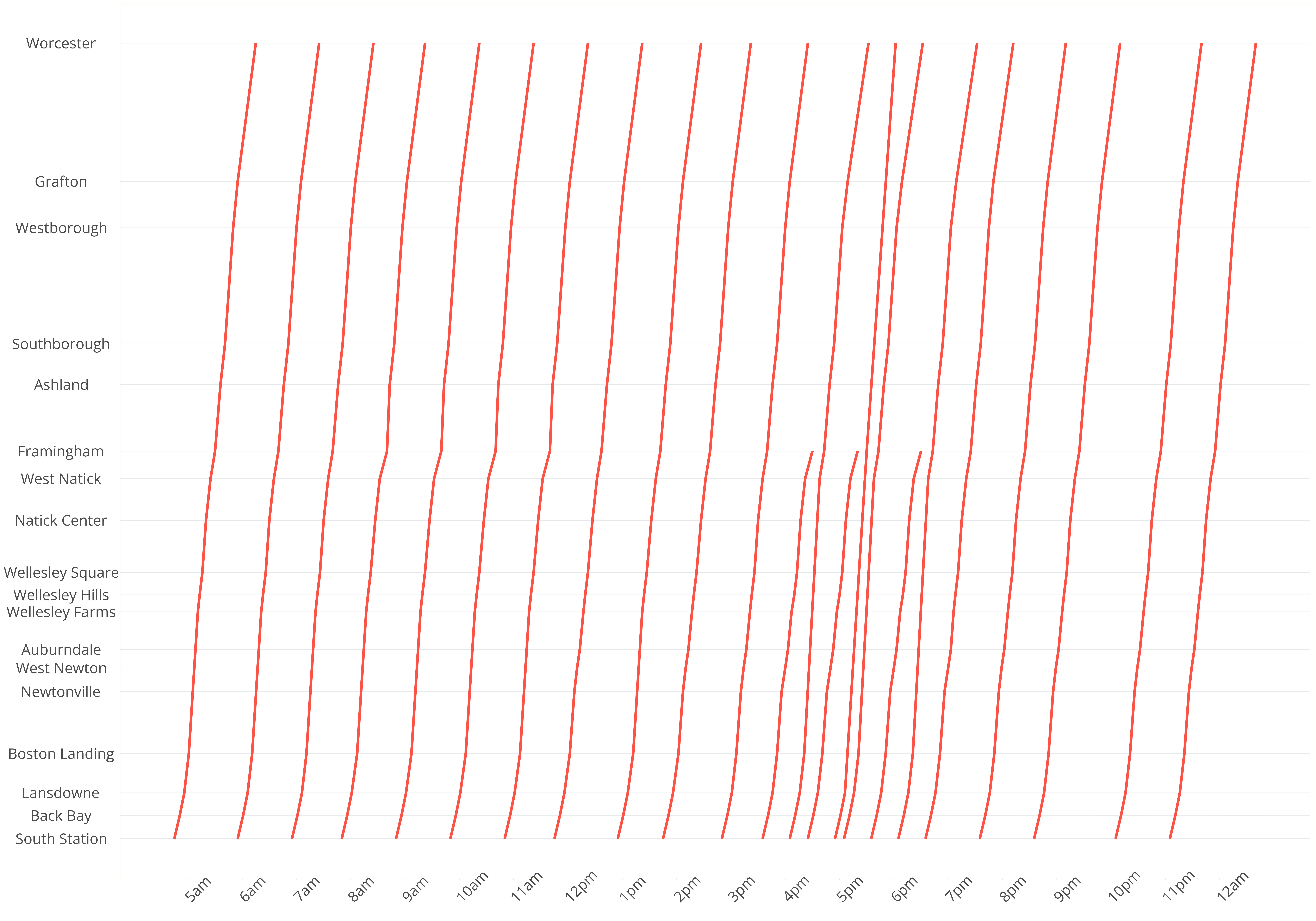
During the first year of the COVID-19 pandemic, the shape of daily travel demand underwent a dramatic shift. While system-wide MBTA ridership initially dropped 90%, peak-period ridership dropped even further, flattening demand throughout the day; many “essential workers” were traveling outside of traditional peak periods based on 9am-5pmwork schedules (Figure 3). The MBTA was forced to figure out how to plan and provide more consistent service that supported midday ridership, which had become a greater percentage of total daily trips.
For years, places like Switzerland have demonstrated the advantages of scheduling structures like regular timetables, or clockface schedules, in which trains depart at the same number of minutes past the hour every hour.4,5 Consistent departure times within each hour make it easier for riders to memorize the transit schedule, which is particularly important for infrequent riders, and may therefore help induce new ridership.6 A 2022 study of Swedish regional public transit demonstrated that increasing frequency outside peak hours increased ridership in both peak and off-peak hours for years after changes wereimplemented.7 This may be because frequent off-peak service can attract travelers by providing more departure times and the additional service provides a sense of security for return trips. Thus, the MBTA’s shift to more consistent all-day service with more memorable train times is consistent with a durable increase to ridership on its Commuter Rail system.
MBTA Commuter Rail ridership historically has more riders that are in higher income brackets and identify as White than other transit services in the system, according to the MBTA System-Wide Passenger Survey.8 The share of riders who identify as Federal Transit Agency-designated “minority” and “low-income” riders has grown across all modes, but notably, these increases have been greatest on the Commuter Rail. Riders of color on the Commuter Rail increased from 15% of ridership in 2015-17 to 38% in 2022. Across the MBTA system, the share of these “minority” riders increased from 34% to 58%.“Low-income” riders on the Commuter Rail grew from 7% of ridership in 2015-17to 26% in 2022, whereas they increased from 29% to 48% of overall ridership. The Commuter Rail schedule change, which occurred in April 2021, can better match the transit demand profile of riders who are often low-income or Black, Hispanic, or Asian. Specifically, the transit literature indicates that lower-income riders, as well as groups who have historically faced discrimination, are more likely to rely on transfers and make more stops on their route, in part, due to lower car ownership rates and a mismatch of transit services.9,10 This pattern bolsters the argument that providing more frequent service is necessary to meet the needs of the most vulnerable and transit dependent users.
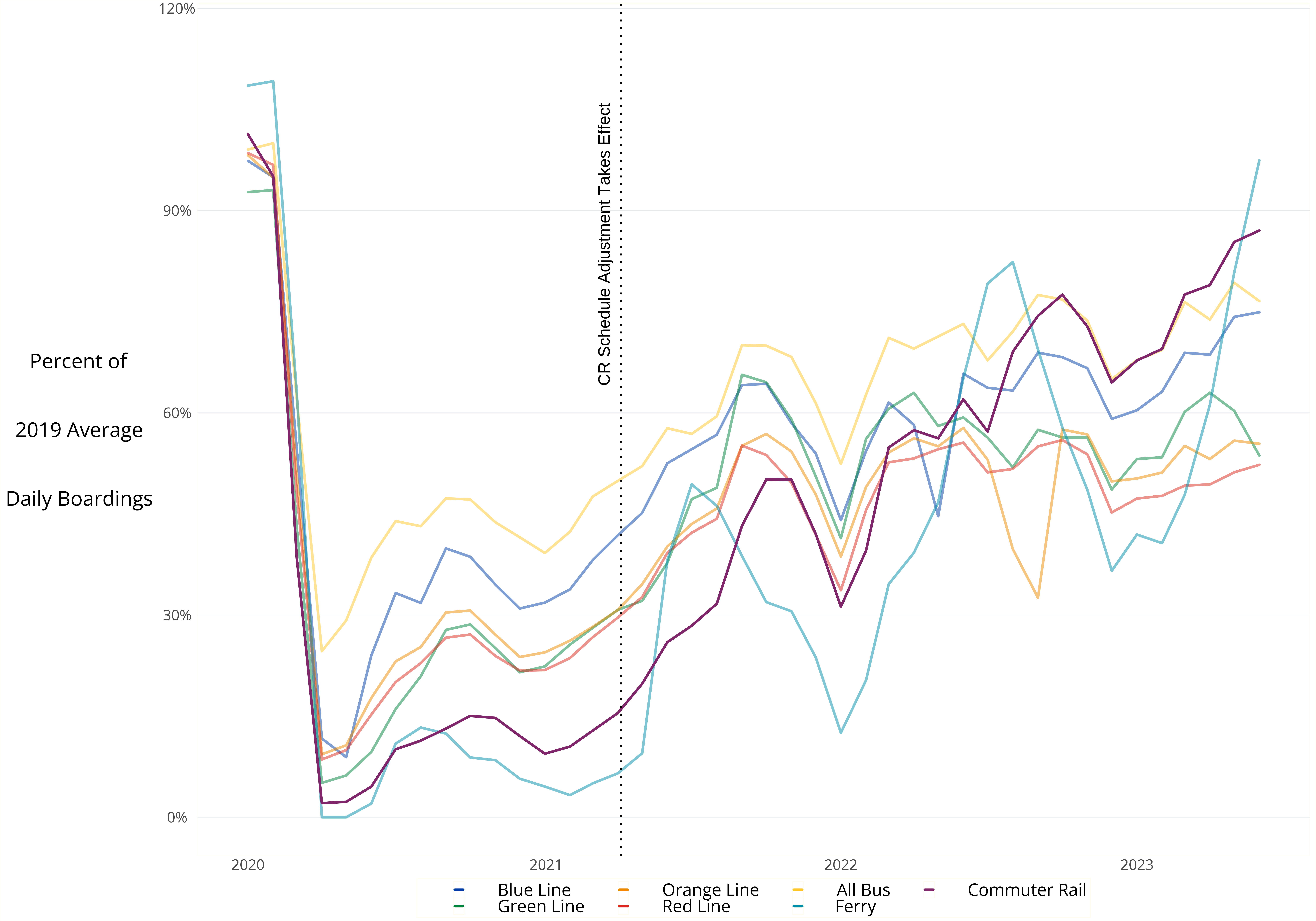
Transit ridership growth following the initial drop in 2020 is due to factors such as the availability of the COVID-19 vaccines, return-to-office policies, and the general acceptance of COVID-19 risks among the public. Based on these changes and evidence from other transit agencies and on other MBTA transit modes, ridership on the Commuter Rail would have increased even in absence of a schedule change. Another factor that likely affected ridership growth is declining rapid transit service on the MBTA. Over the last year, the competitiveness of the MBTA’s rapid transit system compared to other travel modes has also declined due to factors including operator shortages, declining vehicle availability, and poor track condition. Travel times have increased and passengers’ confidence in rapid transit has decreased, which has pushed travelers toward alternative services like the Commuter Rail when available.11 MBTA press releases have specifically recommended using Commuter Rail, where available, as an alternative to disrupted rapid transit services. Evidence suggests that inefficient travel time ratios of rapid transit services relative to other modes reduce their market share.12,13
However, as outlined above, the literature suggests that the schedule changes on Commuter Rail should have caused ridership increases above what would otherwise be expected. The dramatic increase in ridership on Commuter Rail (Figure 4), especially when considering the relatively low ridership gains on the MBTA’s three most heavily used rapid transit lines (Red, Orange, and Green), warrants further exploration. To answer this question, we conducted an analysis of daily boardings to estimate the impact of the schedule updates on Commuter Rail ridership.
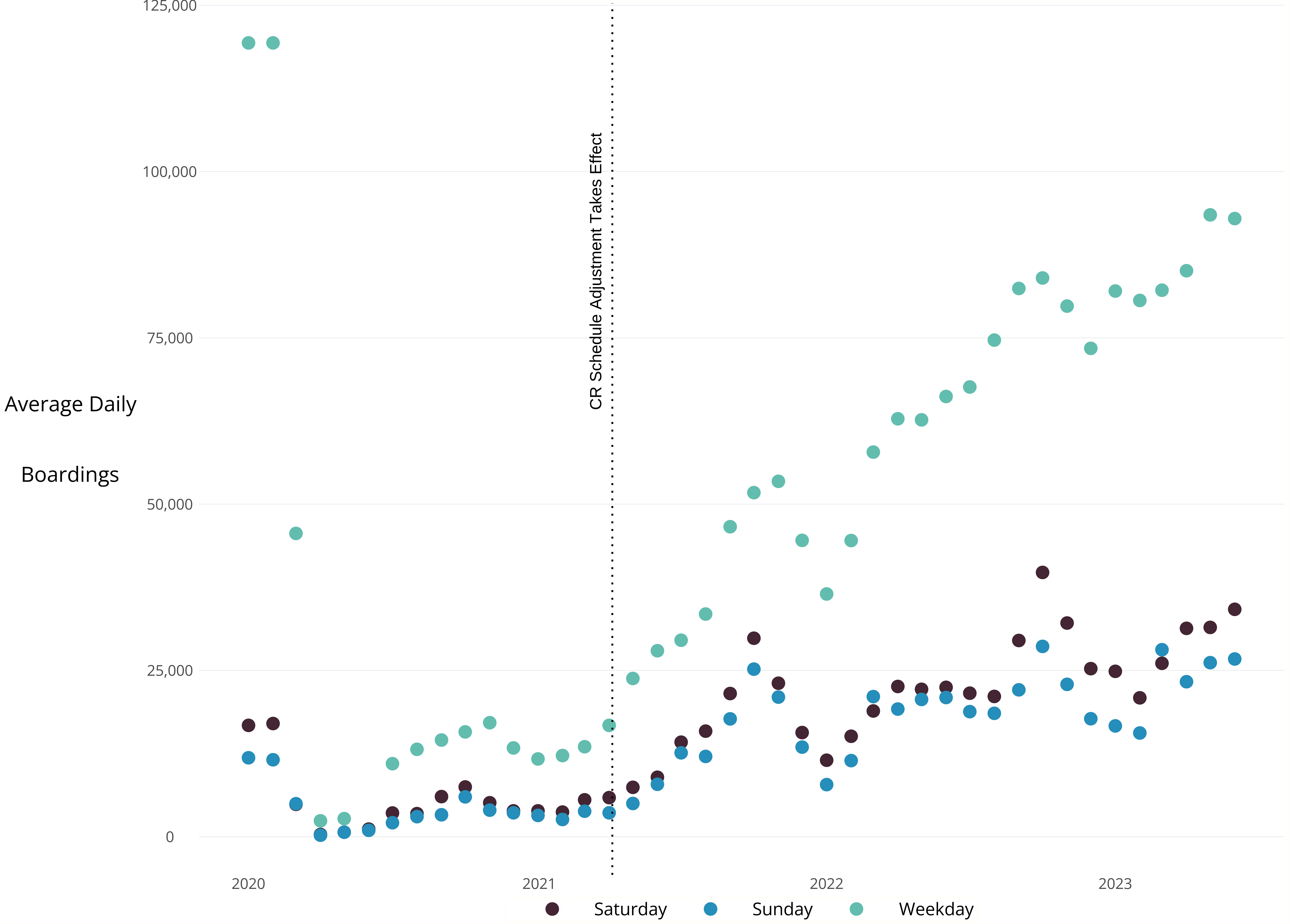
Using a regression analysis to estimate ridership growth from service improvements
Method
We used a multiple linear regression model that controls for other changes to general transit ridership recovery (described above) and declining rapid transit service on the MBTA. Day type was another important consideration in the model as Commuter Rail varies greatly between weekdays, Saturdays, and Sundays. Weekday ridership is higher than Saturday ridership, but daily boardings as a percentage of pre-pandemic levels are higher on Saturdays than on weekdays. We therefore examined the effects of the schedule changes on each day type individually.
General Transit Ridership Recovery. To account for general ridership recovery across the MBTA, regardless of schedule changes, we used the average percentage of pre-pandemic ridership from several of the MBTA’s peer agencies, particularly those which have commuter rail lines and accommodate 9-to-5 rail commuters (Figure 5). For each month, the total ridership for each agency was calculated as a percentage of average ridership in 2019. The average across the six agencies was taken to represent the average percentage.14This percentage served as an indicator for general transit ridership recovery throughout the pandemic and assumed that the recovery on the MBTA’s Commuter Rail would be similar.
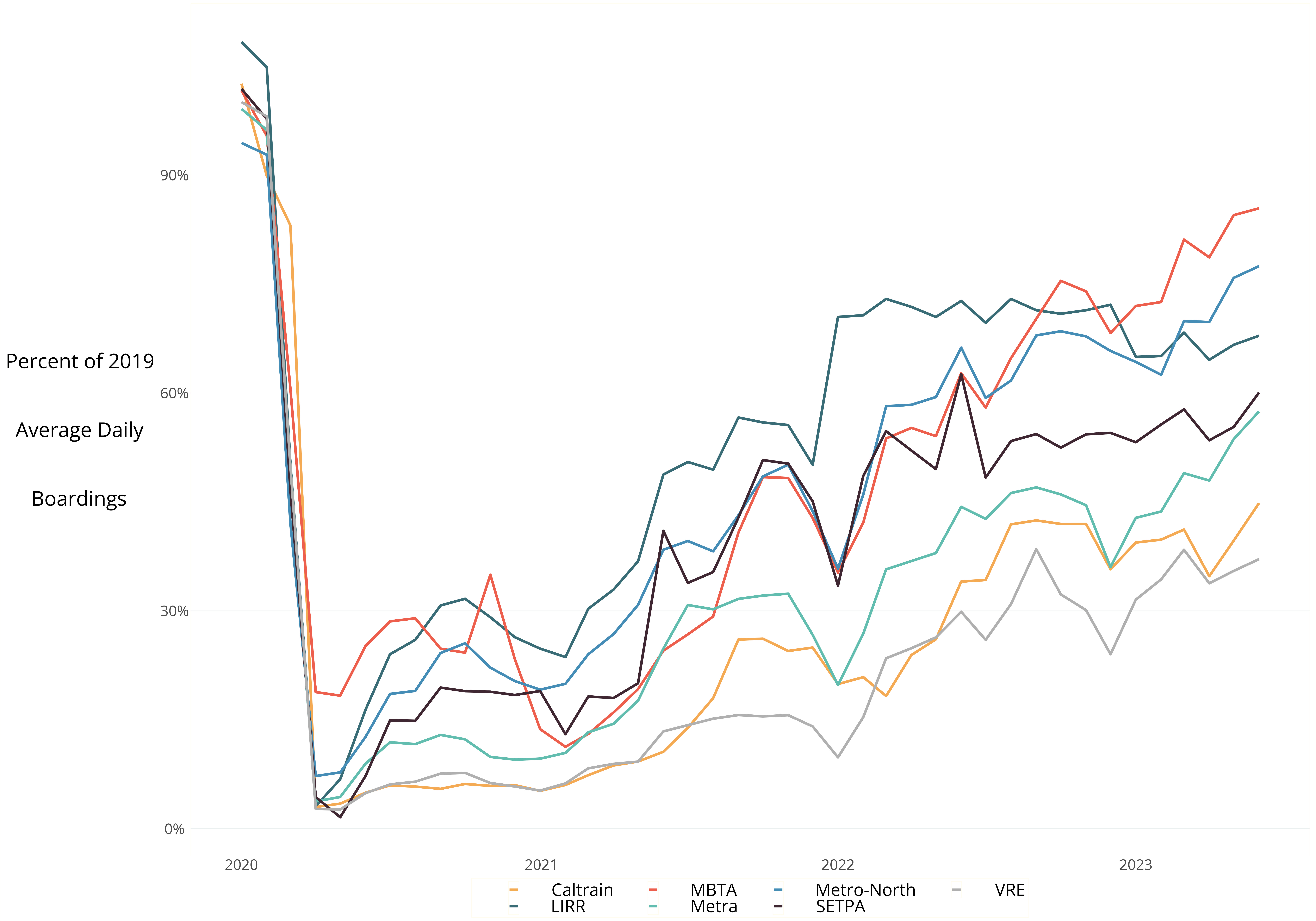
Declining Rapid Transit Service. We used average travel time on heavy rail lines to control for transit service that is substitutable for Commuter Rail. This assumes that worse travel times on service parallel to the Commuter Rail correlates to increased use of Commuter Rail services. Average travel time accounts for periods when service was diverted on parts of heavy rail lines, or even entire lines, causing delays. Ideally, a metric such as excess journey time would serve as a better indicator of competitiveness; this metric is under development at the MBTA. However, because a prominent factor inhibiting the competitiveness of rapid transit at the MBTA over the last couple of years has been speed restrictions greatly increasing average travel times, this metric serves as a proxy, although imperfect, for competitiveness.
Data. Data sources includes daily boardings on the Commuter Rail, which are calculated based on conductor counts on board and manual counts from terminal stations, and MBTA heavy rail rapid transit trip time data, which measures the average time it takes a rider to travel from one end of the Red, Orange, and Blue lines to the other end. Data were analyzed using the R Statistical Software package stats (4.2.2) and its “lm” function for fitting linear models.
Results
Schedule change is significantly associated with a large increase in average daily boardings on both weekdays and weekends, even after controlling for ridership recovery on peer agency transit lines and the decline in service on the MBTA’s heavy rail lines. The effect of the schedule change proves to be much larger for weekend ridership—both in absolute terms and as a share of total daily riders. Clockface schedule changes account for an average boarding increase of:
· Weekday: 8% ; 7,300 boardings
· Saturday: 31% ; 10,700 boardings
· Sunday: 34% ; 9,300
Given that the all-day service change largely affected weekday schedules, the growth in weekend ridership relative to weekday ridership is unexpected. Weekend schedules already ran much more evenly throughout the day, and any service improvements on a weekend line largely occurred in the form of an additional train in the daily schedule. These results may confirm previous research that standardizing the schedules of rail services during the week positively influences demand for weekend service without making other significant service changes. Our model does not account for possible service improvements, such as on-time performance, which could have coincided with the changes to the Commuter Rail schedule. Some research has also speculated that shifting population distributions that occurred in 2020-21 could impact travel patterns.15But, since we face data limitations concerning the composition of weekend ridership—e.g., a lack of survey information from weekend riders—we are unable to dig more deeply into this phenomenon.
General ridership recovery and declining rapid transit service were also positively associated with average daily boardings. For every 1 percentage-point increase in peer agency percent of pre-COVID ridership, we saw an increase of about1,500 weekday boardings on the Commuter Rail; this is effectively an estimate of the anticipated recovery without the schedule shift. Notably, weekend ridership recovered less than 200 boardings for every 1 percentage-point increase in peer agency ridership, which is dwarfed by the 9,000-11,000 daily boardings that are attributed to the schedule change. Additionally, for every minute that the average heavy rail travel time increased, average weekday daily boardings increased by 400, suggesting that riders will self-divert to other modes in the face of decreased quality of service when given an option.
Conclusion
Our first analytical model reveals a significant relationship between the implementation of scheduling improvements and average daily boardings. This relationship is particularly strong for weekend travel, as ridership on the Commuter Rail over Saturday and Sunday in June 2023 is almost double ridership in June 2019. However, we do not have a clear answer for why significant changes to weekday schedules could impact weekend ridership to such an extent and argue for more research into weekday ridership growth relative to weekend ridership growth on Commuter Rail services. This relationship between scheduling and ridership remains a positive sign for the ability of a regional rail model to affect mode share, which is particularly important in an era when agencies seek alternative ways to support riders whose trips are affected by needed repairs to aging infrastructure.
As emphasized in the literature, all-day service offers much greater convenience and reliability to a wider range of riders, in addition to operational benefits that enable more efficient infrastructure planning. The COVID-19 pandemic emphasized that riders with lower incomes or without a car were much more dependent on public transit and that service could not only be designed for those working 9-to-5 jobs.16Service frequency is also one of the most significant policies that can affect transit use, and there is clear evidence of induced demand when trains run frequently.17,18To this point, we can expect that work commuters who were reluctant to use the Commuter Rail because it was only reliable during peak hours may be enticed by improved all-day service. These more legible schedules require less up-front planning for riders since they do not have to pore over timetables to understand when their stop will be served.
Further analysis: modeling equity implications
A second analysis was performed as apart of this paper that explored and identified different types of riders. This model is briefly summarized below. Full method and results are available upon request or can be found in the upcoming full publication in the Transportation Research Record as “A Case Study of Ridership and Equity Implications of All-Day Massachusetts Bay Transportation Authority Commuter Rail Service.”
The second model used a statistical procedure to identify unobserved subgroups within population from patterns of observed variables. Data was sourced from the MBTA Systemwide Passenger Survey.8These data are collected annually by the MBTA to determine who is using the system and which services they are using and aims to capture the diversity of ridership across all MBTA services. A synthetic dataset based on these responses was created to represent a population of Commuter Rail riders and help classify different types of people that use the Commuter Rail based on characteristics like race, ethnicity, gender, access to vehicles, income, and geographical location.
Our second analytical model demonstrates the three dominant profiles of riders who currently use the Commuter Rail system. The daily commuter is the largest group. When combined with the hybrid commuter, who uses the system for work trips but travels less often, commuters make up a majority of ridership. Yet, understanding the third group—general riders—has the greatest equity implications for how the transition to more even all-day service affects minority groups. The analysis of active covariates shows that making less than$75,00 annually and being Hispanic dramatically increases riders’ odds of being a general rider—i.e., traveling for non-work trips and often during off-peak hours. Being under the age of 26 also increases the odds of being a general rider, which is consistent with the assumption that these riders are not as likely to be working full-time jobs, but has further implications for how we identify barriers to accessing mobility services. For example, most general riders are making transfers during their journey and are traveling more than 10 minutes to or from one of their stops. So, while legible timetables will ease the burden of trip planning, do we have services that further distinguish the Commuter Rail system as a regional rail network and boosts mobility between towns and cities?
References
1. MBTA (Massachusetts Bay Transit Authority). (2020). MBTARail Vision (Final Report). https://cdn.mbta.com/sites/default/files/2021-07/2020-02-rail-vision-report.pdf
2. Shantz, A., Casello, J., Woudsma, C., & Guerra, E.(2022). Understanding Factors Associated with Commuter Rail Ridership: A DemandElasticity Study of the GO Transit Rail Network. Transportation ResearchRecord: Journal of the Transportation Research Board, 2676(8), 131-143. https://doi.org/10.1177/03611981221082573
3. Solís, E., Ruiz-Apilánez, B., Moyano, A., Mohíno, I.,& Coronado, J. M. (2023). Towards Sustainable Regional Planning: Potentialof Commuter Rail in the Madrid Urban Region. Applied Sciences, 13(6),3953. http://dx.doi.org/10.3390/app13063953
4. Maxwell, R. R. (1999). Intercity Rail Fixed-Interval,Timed-Transfer, Multihub System: Applicability of the IntegralerTaktfahrplan Strategy to North America. Transportation Research Record:Journal of the Transportation Research Board, 1691(1). https://doi.org/10.3141/1691-01
5. Hartl-Benz, V. (2015). Integrated regular timetableand spatial factors in Switzerland and Austria. Urban Transport 2030 –Mastering Change, The Fifth International Conference, 5-6 November. https://publik.tuwien.ac.at/files/PubDat_244340.pdf
6. Wardman, M., Shires, J., Lythgoe, W., & Tyler, J.(2004). Consumer benefits and demand impacts of regular train timetables. InternationalJournal of Transport Management, 2(1), 39–49. https://doi.org/10.1016/j.ijtm.2004.04.002
7. Hansson, J., Pettersson-Löfstedt, F., Svensson, H. et al.Patronage effects of off-peak service improvements in regional publictransport. Eur. Transp. Res. Rev. 14, 19 (2022). https://doi.org/10.1186/s12544-022-00543-4
8. MBTA. (2023). 2022 MBTA System-Wide PassengerSurvey. [Data set]. Massachusetts geoDOT. https://mbta-massdot.opendata.arcgis.com/apps/MassDOT::mbta-2022-system-wide-passenger-survey-interactive-web-tool/about
9. Lachapelle, U., & Boisjoly, G. (2023). Breakingdown public transit travel time for more accurate transport equity policies: Atrip component approach. Transportation Research Park A: Policy andPractice, 175, 103756. https://doi.org/10.1016/j.tra.2023.103756.
10. Lubitow, A., Rainer, J.,& Bassett, S. (2017). Exclusion and vulnerability on public transit:experiences of transit dependent riders in Portland, Oregon. Mobilities, 12(6),924-937. https://doi.org/10.1080/17450101.2016.1253816
11. MBTA (2020-2023). Customer Opinion Panel. https://mbta-massdot.opendata.arcgis.com/datasets/mbta-service-customer-satisfaction/explore.
12. MassINC (Massachusetts Institute fora New Commonwealth). About the Gateway Cities. [cited 2024 April 7] https://massinc.org/our-work/policy-center/gateway-cities/about-the-gateway-cities
13. Liao, Y., Gil, J., Pereira, R. H. M., Yeh, S., & Verendel, V. (2020). Disparities intravel times between car and transit: Spatiotemporal patterns in cities. ScientificReports 10, 4056. https://doi.org/10.1038/s41598-020-61077-0
14. Federal TransitAdministration (2023). National Transit Database: Complete Monthly Ridership(with adjustments and estimates). https://www.transit.dot.gov/ntd/data-product/monthly-module-adjusted-data-release.
15. Chiumenti, N. (2021). How theCOVID-19 Pandemic Changed Household Migration in New England (RegionalBrief 2-3). Federal Reserve Bank of Boston. https://www.bostonfed.org/publications/new-england-public-policy-center-regional-briefs/2021/how-the-covid-19-pandemic-changed-household-migration-in-new-england.aspx#collapse2
16. Palm, M.,Allen, J., Zhang, Y., Tiznado-Aitken, I., Batomen, B., Farber, S., &Widener, M. (2022). Facing the future of transit ridership: shifting attitudestowards public transit and auto ownership among transit riders during COVID-19.Transportation. https://doi.org/10.1007/s11116-022-10344-2
17. Taylor, B.D., Miller, D., Iseki, H., & Fink, C. (2009). Nature and/or nurture?Analyzing the determinants of transit ridership across US urbanized areas. TransportationResearch Part A: Policy and Practice, 43(1), 60-77. https://doi.org/10.1016/j.tra.2008.06.007
18. Chakrabarti,S. (2017). How can public transit get people out of their cars? An analysis oftransit mode choice for commute trips in Los Angeles. Transport Policy, 54,80-89. https://doi.org/10.1016/j.tranpol.2016.11.005